Do technical mechanisms for building ‘trust’ help people trust systems?
November 21, 2020
7 min read
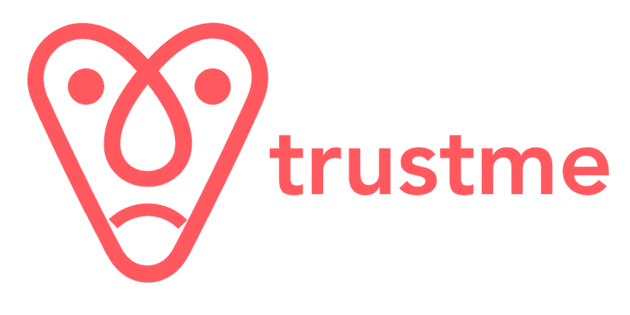
Technical mechanisms for building trust do help people trust systems - the rise of e-commerce sites and in particular sharing economies is in large part due to the mechanisms the platforms use to address trust concerns between the two parties. However, these trust mechanisms break down if the users feel there is a lack of transparency, or if they feel the system is no longer acting in their best interests.
Whenever two parties participate in a transaction, each party must trust that the other party will uphold their side of the bargain. However these transactions are often made on the basis of incomplete information about the other party. Trust mechanisms are used to provide signals about the other party to reduce the asymmetry of information. Platforms use trust mechanisms to reduce users’ risk.
For example, Apple’s security and review mechanisms around the App Store facilitates the installation of third-party iOS applications. The vetting of apps submitted to the App Store signals a minimum level of quality, whilst the sandboxing of apps when run guarantees the security of the user. This means that users no longer have to rely on whether a third-party vendor was reliable. Their trust in Apple, the platform provider, means they are willing to install third-party apps from vendors they haven’t heard of.
The most prominent example of a trust mechanism is a ratings or review system. Apps and online products are a “market of lemons” as, aside from the brands with existing reputations, customers cannot distinguish the quality of products. Customers therefore do not trust the system, as they anticipate sellers could profit from selling low-quality cheap items (“lemons”). Ratings and reviews serve to filter out the “lemons” in the market, and emulate word-of-mouth networks to increase trust amongst users. The market forces now disincentivise lemons as deceitful sellers’ short-term gains are outweighed by the long-term loss of reputation.
Amazon’s recommender systems achieve a similar result: here the platform (the institution) filters out low-quality items, and ranks the products in order of relevance to the user, rather than the user having to sift through reviews. These ratings and recommender mechanisms have a ripple effect in terms of trust over the entire system. Zhao et al. show that buyers’ trust in these institutional mechanisms “engender trust, not only in a few reputable sellers, but also in the entire community of sellers”.
Trust mechanisms play an even bigger role in sharing economies, such as Airbnb and Uber, where the level of trust required between consumers and providers on the platforms is especially high, as sharing economies offer services not products. Services are “intangible goods [whose] quality cannot be verified”, and offer additional risks (not just monetary), for example a reckless Uber driver could endanger the passenger. Hawlitschek et al describe that trust is composed of 3Ps - peer, platform (and product) – which “positively influence consuming (and supplying) intention”. Airbnb ensures trust in the platform through dependability - maintaining a high system quality. In order to get consumers to trust the providers, Airbnb shows the person behind the service, by getting hosts to upload photos and show the guests the living space. The photo also acts as a means of identity verification. Ert et al argue that this “visual-based trust” affects the consumers’ perception of hosts as much as previous ratings. Botsman describes how Airbnb and Uber’s rating mechanisms facilitate a notion of distributed trust between users of the platform. Two-sided reviews incentivise good behaviour for both consumers and providers, since users have a reputation to preserve, as the ratings affect future interactions on the platform. Whilst there is potential for users to game the system, these platforms have mechanisms in place to mitigate against these. Airbnb has double-blind reviews to prevent guests and hosts from writing retaliatory reviews. Finally, Airbnb offers payment protection for the consumer, and insurance protection for the host, reducing their financial risk. Möhlemann finds that (as with e-commerce sites) this protection, simultaneous reviews and large network increased the trust in the platform provider, and this trickled down to increased trust in the peers.
Trust mechanisms aren’t perfect however, as bad actors can manipulate the trust mechanisms by posting false reviews, especially when the seller is new to the market and doesn’t have a reputation to preserve. Which? found that almost 90% of 12,000 reviews for headphone products were from unverified consumers. Even good actors can bias the ratings mechanism upwards, as they fear the threat of retaliatory feedback, leading to inflation of reviews. These mechanisms aren’t just manipulated by users of a platform but the platform itself - treating the trust mechanism as a public good, Amazon can free-ride off third-party sellers and use the ratings to determine which product categories are successful, and enter them themselves.
More concerning side effects of trust mechanisms include discrimination. Airbnb’s policy of requiring hosts and guests to upload photos has led to a “dizzying array of stories” about racial bias exhibited by hosts, violating the Civil Rights Act of 1964: Title II – Public Accommodation. Here, the issue is that in the process of attempting to build trust, the trust mechanism revealed protected characteristics (race, gender) of the users of the platform, opening the platform up to discrimination. Airbnb has since addressed this with an updated anti-discrimination policy, which was praised for its speed and transparency by the CEO of the Leadership Conference on Civil and Human Rights.
In the case of Uber, Hanrahan et al. found that the ratings mechanism used was too crude and lacked a “human in the loop to impart a level of flexibility or subjectivity to the process” and that the rating system failed to capture the nuance and reasoning behind a poor review. They argue that the digital infrastructure powering the Uber platform potentially reinforces and propagates the sociodemographic biases present in users, and these poor ratings assigned to drivers go on to affect future service requests. However, the key difference between Airbnb and Uber was the lack of transparency regarding the rating system. This led to suspicion amongst drivers of racial bias and a lawsuit filed against Uber for racial discrimination regarding the firing of minority drivers with low ratings. The ratings mechanism in this scenario propagated mistrust, as frustrated drivers posted retaliatory poor ratings for passengers.
The lack of transparency in the ratings system also belies a lack of accountability, which fosters mistrust in the system. This is a pattern that has permeated more broadly in tech companies. The Edelman Trust Barometer Survey found that consumers “report high levels of “weak trust,” which means while they remain trusting, they do so with significant misgivings”. The survey further states that only 39 percent of respondents in developed markets believe tech is putting the welfare of its customers ahead of profits.
Herein lie the limitations of technical mechanisms in fostering trust in a system. Mayer et al. state that organisational trust is composed of three factors: ability (the capability of a trustee to accomplish a trust goal); benevolence (the extent to which the trustee is believed to want to do good to the truster) and integrity (the perception that the trustee adheres to a set of principles the trustor finds acceptable). Moreover, Mayer et al argue that the effect of perceived benevolence plays a larger role in users’ trust as their relationships with the system become more established. Technical mechanisms can only improve the ability of a system, but cannot address the perception of benevolence or integrity.
Technical mechanisms of improving trust typically seek to produce signals as a proxy for the private data the other party has not divulged. In the case of recommender and ratings systems, this typically comes from an aggregation of huge swathes of users’ personal data. The same mechanisms that sought to address the asymmetry of information have become so good at aggregating data that users find them “creepy” . Again, the lack of transparency around recommendations leads users to distrust the platforms, accusing them of “listening in on their conversations”. Earlier in 2020, Airbnb acquired a patent for AI to perform a background check on guests, predicting personal traits from social media accounts. However this damages the third pillar of trust - integrity - as this level of automated inference using personal information violates users’ principles regarding privacy. Indeed, here the perceived increase in trust due to better guest vetting is significantly outweighed by the damage of integrity. In the case of Amazon, the increasingly personalised recommendation systems lead to users believing that the system is optimising for the platform’s profits over providing better-value recommendations to users, damaging the perceived benevolence of the brand.
The increased automated decision-making as a result of these technical mechanisms “change the nature of trust produced by these institutions” according to Bodó. Bodó argues that even if these technical mechanisms do “no more than formalize and encode internal rules and procedures”, the removed human discretion will lead to the inability to adjust for exceptions or interpretations. This lack of nuance could lead to incorrect decisions that damage users’ trust, for example if Uber bans innocent drivers from its platform. Furthermore, the lack of transparency means that these mechanisms lack accountability, and, as Uber demonstrates, contain unchecked implicit algorithmic bias. Therefore, in their current state, for large established systems, technical mechanisms no longer improve trust in the system.
The potential way forward for these technical mechanisms to continue to improve trust in the overall system is through increased transparency . This is non-trivial, as Lawrence argues there is an intellectual debt associated with these systems . The implementers of a system do not understand the machine learning algorithms used - simply exposing the source code would not be sufficient to solve this issue. Moreover, this push for increased transparency might also conflict with privacy and also make these trust mechanisms more susceptible to being manipulated by bad actors.
Likewise, addressing bias in the system does not have a clear-cut technical solution. Defining a notion of fairness is not straightforward as there are multiple statistical notions of fairness, and Courtland shows that these are often in conflict with one another. Therefore, Courtland argues that these statistical mechanisms of ensuring fairness will only “ameliorate bias but not eliminate it”.
Ultimately, technical mechanisms for improving trust do result in an increased user trust in the system, until users no longer trust the technical mechanisms themselves. Despite the public shift in perception regarding privacy and the power of tech platforms following the Cambridge Analytica scandal, it is important to underscore that trust mechanisms form the bedrock of trust in e-commerce and sharing economies, as they compensate for the increased asymmetry of information present in the virtual world compared to the physical world. However, as these platforms grow, there is an increased power imbalance between the platform and users of these platforms. Coupled with the lack of accountability and transparency behind these mechanisms, this has led to a distrust in the platforms themselves, even if their trust in the service provided by these platforms is unchanged.
Technical solutions to addressing bias and transparency are insufficient, as they lack the nuance to handle exceptional cases, hence the need for human oversight of these systems. Given the incentives for platforms like Amazon to manipulate trust mechanisms for strategic competitive advantages, this will likely need to come in the form of external regulation. In this scenario, the main factor in increased user trust will be through regulation like GDPR, as it addresses users’ concerns regarding privacy and data protection. This regulation will help realign the system’s principles with the users, increasing its perceived integrity and its trust.